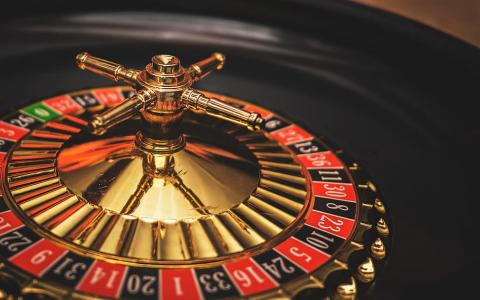
Technological disruption has reached the investment markets. You can either follow the lead of people like Cathie Wood and load your client portfolios with machine learning stocks . . . or actually deploy these systems alongside pioneers like Beaumont Capital to provide more robust overall client outcomes.
Think back to the familiar Monte Carlo simulation. You take the historical “deck” of annual market outcomes and deal out every possible hand for your clients.
Add up the score to show them the odds of beating Wall Street’s random walk.
It isn’t predictive. It’s for illustrative purposes only. Right?
But computers that can deal every possible hand can also spot emerging patterns. When the cards start stacking up against your client’s portfolio, they can search through market history to suggest a better alternative.
That’s machine learning. With enough “experience,” an algorithm can now weigh the odds, gauge the market weather and anticipate sweet spots . . . while dodging obvious pain points.
Beaumont Capital Management is ahead of the game in training its algorithms. I talked to their machine learning guru Brendan Ryan a few days ago and he’s willing to talk to you too.
The Experience Of A Lifetime
Traditionally, every “game” of Monte Carlo we play for a client constructs a range of possible lifetimes. Some will be great, leaving a lot of money behind. Others will be miserable.
Most will be somewhere in the middle. The simulation’s only job is to determine where the odds will give the client enough comfort to trust the financial plan.
However, each client only gets one real deal of the cards. Everything else is simulated.
A computer program, on the other hand, can play out a vast number of “games” or hypothetical market timelines. The more it plays, the better it gets at spotting common errors and capturing opportunities that would otherwise be ignored.
Play enough games and the algorithm has “seen” it all. While it can still be surprised by unprecedented market conditions (a pandemic or a credit crash, for example), its library of responses improves to the point where it can recover faster than most human investors.
Brendan Ryan tells me the Beaumont algorithms faltered a little like the rest of us in the initial pandemic bear market last year, but soon recognized that the economy was splitting into the now-famous “K” formation.
Some stocks were safe to buy on the dip and Beaumont models bought them. Others, on the downward leg of the “K,” remained off limits.
It took human investors over a month to figure that out. If they bailed out on the way down, they were late to get their clients back in. And if they simply froze, they’re still holding a lot of stocks that never recovered.
And the Beaumont algorithms are still learning. The pandemic added a few unprecedented cards to the old Monte Carlo deck, forcing the system to deal them into its simulations to find strategies that could cope.
That’s the thing about machine learning. It gets better as you introduce new data. Investors whose advisors parked their money in Beaumont portfolios are unlikely to ever suffer a pandemic shock again.
Pandemics may come and go. But the algorithms have already dealt that card and know now who wins and who loses. They’ll build the best possible hand out of the deck the future provides.
Mortal Versus Machine
The system even compensates for human error. Brendan Ryan tells me Beaumont has spent a lot of effort “training” its tools to recognize and exploit scenarios where investors fold under stress.
It’s easy to think of examples. When a hedge fund runs out of luck and needs to make payroll, its liquidation follows broad patterns. Spot the patterns and buy the dip.
Interestingly, we didn’t talk about what the algorithms do when they see irrational exuberance hitting a wall. But I suspect that’s coming . . . and if you want to get your clients ahead of the curve, Beaumont is happy to help.
While they appreciate the downside protection, they’re more interested in picking up easy wins by reading the tells of other investors losing their nerve.
As Brendan Ryan puts it, loss aversion creates its own myopia. Great companies tend to remain great investments over time, but when people lose sight of that, they tend to fold just like those hedge funds trying to scrape up payroll.
The algorithms don’t run on human parameters. They never fold a winning hand and will pivot the moment they recognize a threat in the air.
From their endless simulations, they can see black swans coming. But unlike human managers, they don’t dodge a black swan that isn’t actually there.
And they get better and better over time. Beaumont invested early in its system. At this point, the algorithms have had time to learn from all of market history . . . plus the pandemic and its aftermath.
They’ve dealt every card in the Monte Carlo deck and will pick the best hand from the factors that prevail in the market, day by day.
That’s the kind of edge that only widens over time. The earlier investors start taking advantage of it, the stronger the ultimate outcomes can get.
Of course, we’re only scratching the surface. It's a lot more than just chasing machine learning stocks like Cathie Wood does in an effort to keep ahead of change.
But if you’re interested in hearing how to deploy change on your clients' behalf, Brendan Ryan will give you all the details.